Utilizing Machinery Breakdown Insights for the Pulp & Paper Industry
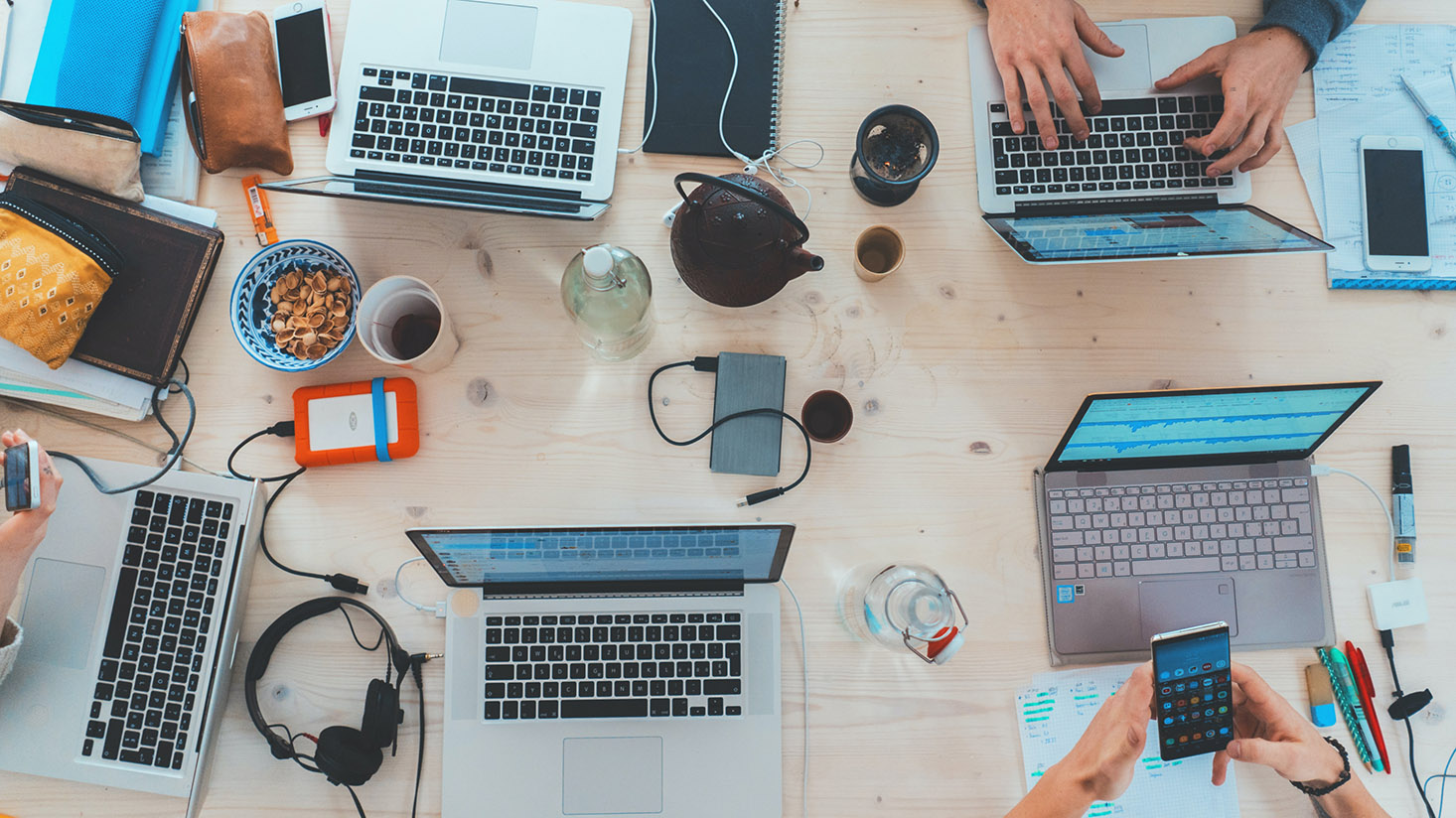
Introduction
The world of manufacturing has always been driven by machines, their efficiency, and their output. However, understanding the intricacies of machinery breakdown, especially in industries such as pulp and paper, has often remained elusive. Traditionally, gaining insights into such breakdowns relied upon manual reports, maintenance logs, and anecdotal evidence. Before the advent of sophisticated data systems, companies were in the dark, waiting months for comprehensive assessments of machinery failures. This disconnect from real-time insights posed significant challenges in decision-making, operational efficiency, and financial forecasting.
Historically, firms might have utilized rudimentary logs or isolated repair incidents to piece together an understanding of machinery breakdowns. These older methods, relying heavily on paper records and manual inputs, were time-consuming and prone to errors. In the days before sophisticated data analytics, companies relied on physical inspections and sporadic maintenance records compiled over years. The lack of centralized and actionable data often meant businesses could not react swiftly to breakdown incidents, resulting in longer downtimes and increased costs.
The leap from antiquated methods to current data-driven approaches can be attributed to technological advancements. With the rise of the internet, sensors, and connected devices, collecting and analyzing machinery breakdown data has transformed industries. These innovations have enabled the storage of every operational blip, creating detailed databases that deliver real-time updates. As a result, firms are no longer in the dark about the operational statuses of their machines; they can now predict, react, and optimize operations with unprecedented precision.
The importance of categories of data in understanding machinery breakdowns cannot be overstated. Data empowers businesses to track, analyze, and anticipate machine performance and operational anomalies. This transformation allows operations management to reduce downtime, minimize financial losses, and improve safety standards. Access to real-time breakdown indicators means immediate remediation steps can be undertaken, streamlining operations and fortifying productivity.
Before the digital revolution in manufacturing, businesses were often left waiting for machine updates, plagued by inefficiencies and extended downtimes. The introduction of comprehensive datasets changed this paradigm, offering insights into machinery operation that were previously unattainable. Addressing potential breakdown instances proactively became a hallmark of competitive advantage.
In conclusion, understanding machinery breakdowns in the pulp and paper industry has become integral for sustainable operations and competitive standing. With the sophisticated collection and analysis of data, companies can not only understand past failures but anticipate future challenges, providing a forward-looking approach to operational management.
Financial Data
In understanding the intricacies of machinery breakdowns, financial data plays a crucial role. This data encompasses the financial footprint of machinery operations, providing an expansive view of costs associated with breakdowns and repairs. Historically, financial data regarding machinery was limited to expenditure reports and financial summaries, often captured on monthly or quarterly bases. Today, this type of data has become more granular, capturing machine-associated costs in real-time.
Financial datasets offer an in-depth look at the expenses tied to machinery maintenance and breakdowns. This category of data includes variables such as repair costs, downtime financial loss, and investment in preventive maintenance. Additionally, with external data integration, companies can compare their financial metrics against industry benchmarks, gaining insights into efficiency and competitiveness.
Various industries and roles have leveraged financial data to understand machinery breakdowns better. Financial analysts, operational managers, and strategic planners frequently utilize this data to optimize budgets and justify capital investments in new machinery. The pulp and paper industry, with its reliance on costly and complex machinery, finds financial data indispensable for aligning economic goals with operational needs.
The acceleration of financial data collection has been driven by technology. Advanced enterprise resource planning systems, IoT devices, and real-time data feeds have redefined how financial information is garnered and interpreted. Firms can now monitor machinery-associated financials down to the minute, offering precision in managing machinery-driven budgets.
Specifically, financial data aids in learning about machinery breakdowns in numerous ways:
- Cost Evaluation: Assesses repair vs. replacement costs to make informed financial decisions.
- Budget Allocation: Allocates funds accurately for maintenance and upgrades.
- Risk Assessment: Identifies financial risks due to potential breakdowns.
- Operational Efficiency: Analyzes cost-efficiency of operational strategies.
- Breakdown Insurance: Guides decisions on insurance coverage for machinery breakdowns.
In essence, AI and predictive analytics within financial data realms allow companies to preemptively address breakdown risks, fortifying their bottom line while enhancing operational stability.
Risk Data
Risk data is invaluable for those seeking to understand the probabilities and impacts of machinery breakdowns, especially in the pulp and paper sector. This type of data is historically rooted in risk assessments and insurance records, which document past incidents and associated consequences. While these records were once stored in physical files, they have now transitioned into comprehensive digital databases.
In essence, risk data is a repository of historical failure instances, their root causes, and consequences. By analyzing this data, companies can discern patterns and identify potential risk factors impacting machinery reliability. It serves as a diagnostic tool, enabling organizations to create informed safety protocols and contingency plans to mitigate future breakdowns.
Industries across the spectrum rely on risk data to manage machinery operations proactively. Insurance companies, operational managers, and risk analysts actively use this data to understand and minimize machinery breakdown exposures. For the pulp and paper industry, reducing unforeseen breakdowns is critical to maintaining streamlined production processes and cost-effective operations.
The advancements leading to the acceleration of risk data include improvements in data collection methods, machine learning algorithms, and data visualization technologies. These advances allow organizations to simulate various breakdown scenarios and assess their impacts. Automated monitoring tools further enrich this data, enabling instant alerts when potential risks are detected.
In the context of machinery breakdowns, risk data provides essential insights:
- Failure Patterns: Recognizes recurring failure patterns to suggest preventive measures.
- Risk Modeling: Develops predictive models to assess future breakdown risks.
- Sensitivity Analysis: Analyzes the sensitivity of machinery to different risk factors.
- Safety Protocols: Guides the creation of robust safety and maintenance protocols.
- Insurance Insights: Offers in-depth insights for crafting comprehensive insurance policies.
As organizations continue to accumulate and analyze risk data, they foster a more robust approach to machinery management, preserving operational integrity and safeguarding profits.
Manufacturing Data
The evolving landscape of manufacturing data has transformed how industries understand machinery breakdowns. This data encapsulates detailed operational records, maintenance logs, production rates, and more. Historically, manufacturing data was confined to siloed databases or even handwritten logs, making comprehensive analysis challenging.
Manufacturing data today extends well beyond static logs, incorporating real-time updates from connected devices and advanced monitoring systems. These data points provide a dynamic view of every machine's operational status, making it easier to diagnose potential issues and implement timely interventions.
This category of data is essential for plant managers, engineers, and operational leaders across industries. The pulp and paper industry, with its reliance on vast and intricate machinery systems, gains significantly from leveraging manufacturing data. It allows professionals to maintain machinery efficiency, reduce downtime, and optimize production cycles.
Technological advancements have amplified the reach and utility of manufacturing data. From IoT-enabled sensors to cloud-based analytics platforms, technology aids in collecting, storing, and analyzing far larger and more granular datasets than ever before. This access to data paves the way for predictive maintenance, allowing companies to foresee potential issues before they culminate in breakdowns.
In practice, manufacturing data aids understanding of machinery breakdowns through:
- Real-Time Monitoring: Provides continuous monitoring to detect anomalies.
- Historical Analysis: Reviews past performance to identify weak points.
- Predictive Maintenance: Predicts maintenance needs before failures occur.
- Efficiency Optimization: Optimizes machinery settings for improved efficiency.
- Downtime Reduction: Analyzes downtime events to implement reduction strategies.
Within the manufacturing sphere, such insights translate into operational excellence, reinforcing a company's market position by minimizing unexpected disruptions and maximizing production potential.
Conclusion
This exploration of machinery breakdown data within the pulp and paper industry highlights the integral role data plays in transforming operational insights and strategic direction. Having access to diverse datasets enables businesses to gain a comprehensive understanding of machine operation dynamics, breakdown patterns, and more. This breadth of knowledge equips business professionals to make sound, data-backed decisions that drive efficiency and resilience.
In today's data-driven economy, it has become increasingly crucial for organizations to leverage different types of data—financial, risk, manufacturing, and beyond. Emphasizing strong data discovery practices empowers companies to refine their operational strategies, maximizing productivity and minimizing disruptions. By becoming more data-driven, firms can transform potential risks into strategic opportunities, fortifying their competitive edge in the market.
Data monetization is an emerging trend that sees companies capitalizing on insights gleaned from proprietary datasets. For businesses, especially those in manufacturing, the ability to extract value from historical machinery data represents untapped growth potential. Strategic data usage could potentially open up revenue streams, driving innovation and informing future operational protocols.
The future promises even more sophisticated data types emerging from technological advancements. From enhanced predictive analytics that preempt machinery failure to complex AI-driven decision models, companies will likely see unprecedented opportunities to harness data for operational excellence. The continued democratization of data and analytical tools will further empower organizations to efficiently, effectively, and proactively manage machinery operations.
As corporate entities continue to embrace data-centric paradigms, the capacity to analyze and extrapolate from extensive datasets will increasingly determine industry leadership. Companies must prepare for this future by investing in data acquisition, storage, analysis, and strategy, fostering a culture where data insights are seamlessly integrated into decision-making processes.
Appendix
Numerous industries and roles benefit from machinery breakdown data. For consultancy firms advising manufacturing entities, having expansive datasets allows tailored solutions and informed consultancy. Insurance providers assessing policy risk can offer more competitive premiums with precise data insight into breakdown frequencies and severities.
Investors relying on this data can gauge operational risks within target companies, making better investment decisions. Accurate insights into machinery operation reinforce investor confidence, potentially increasing valuations and strengthening financial portfolios. Additionally, operational managers leverage data to define process optimizations and reduce breakdown occurrences.
Manufacturing consultants often employ such data to understand client needs deeply and advocate for operational improvements. Collaboration with client teams fosters a proactive approach to maintenance and shutdown planning, improving production outcomes. Risk data, in particular, provides essential insight in creating robust plans to safeguard against potential operational disruptions.
AI continues to offer groundbreaking potential in uncovering hidden insights. By utilizing decades-old documents alongside modern analytics, AI applications could reveal patterns and recommendations previously inaccessible. As advancements in machine learning and AI show continual promise, they enhance the potential to drive innovations within machinery maintenance industries.
The convergence of AI and predictive analytics in the near future is expected to yield intelligent systems capable of autonomously managing machinery health. These systems, fueled by comprehensive datasets, will predict failures long before they occur, ensuring seamless transitions and consistent operational efficacy.
As industries recognize and embrace the power of tapping into expansive, diverse data pools, they dynamic landscape of machinery management is poised to experience revolutionary change. In bridging historical data reliability with innovative trends, companies can craft visionary strategies, ensuring sustained growth and industry leadership.