Whole Slide Digital Images Data
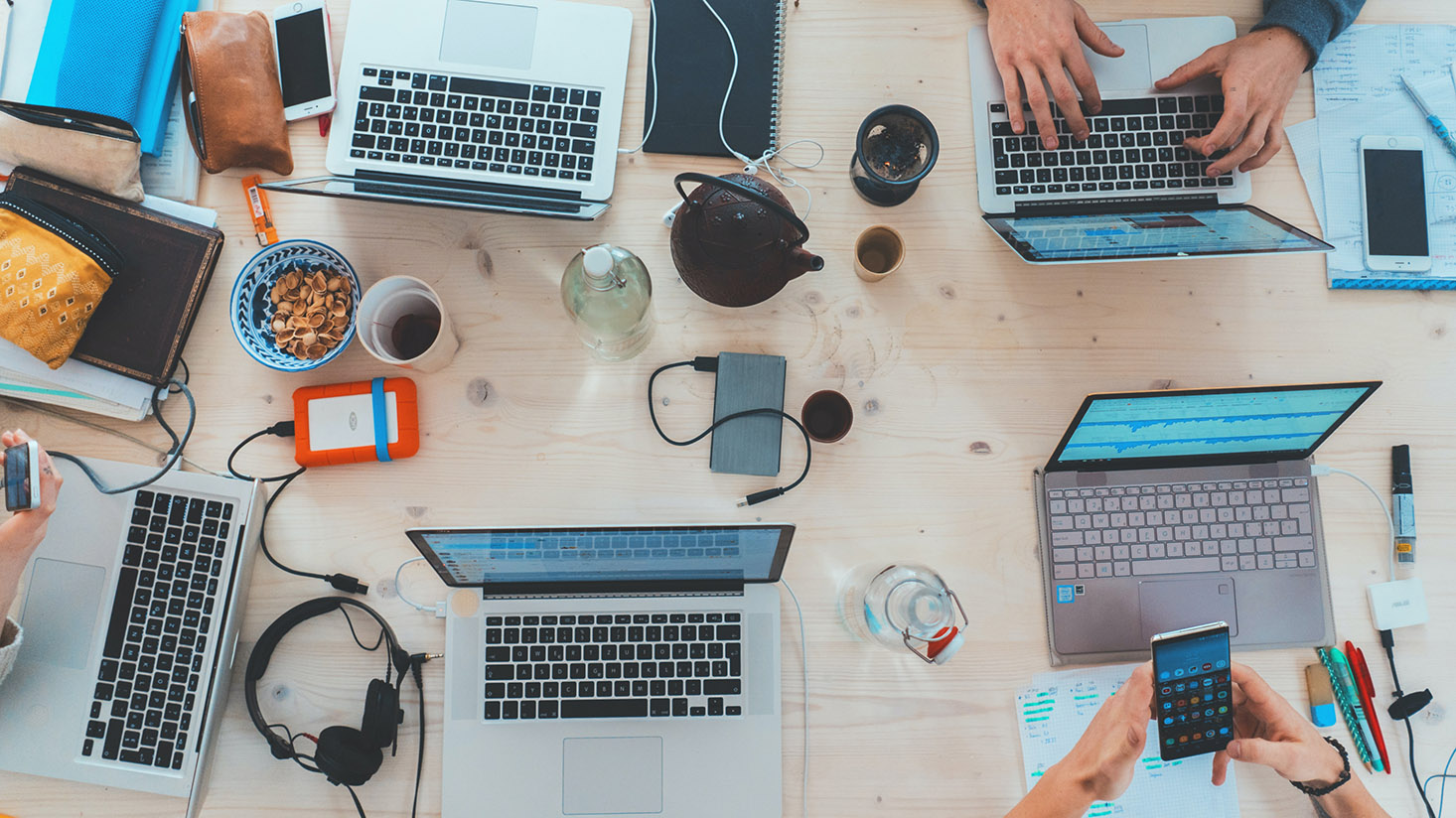
At Nomad Data we help you find the right dataset to address these types of needs and more. Submit your free data request describing your business use case and you'll be connected with data providers from our over
partners who can address your exact need.
Thank you! Your submission has been received!
Oops! Something went wrong while submitting the form.
At Nomad Data we help you find the right dataset to address these types of needs and more. Sign up today and describe your business use case and you'll be connected with data vendors from our nearly 3000 partners who can address your exact need.
Data sets and AI technology have become increasingly important for understanding and optimizing the insights possible from whole slide digital images. Datasets have proven to be invaluable in helping professionals, including medical professionals, to better interpret and interpret digitized whole-slide images. AI-based algorithms and techniques have become ubiquitous in healthcare, allowing for automated image analysis for both healthy slides and slides with a particular illness or disease. In order to understand and make decisions about a whole slide image, AI Training Data and Healthcare Data should be leveraged.
Whole slide digital images are invaluable for healthcare professionals in terms of diagnosis and predicting a particular illness or disease. However, due to the fact that the features of the images are generally too small to be seen at a zoomed out scale, this task has been made more difficult over the years. AI and training datasets have made it possible to extract the relevant features of a whole slide image, even at a low resolution, to enable a more comprehensive analysis of these images.
To analyse and make meaningful predictions regarding a particular image, AI Training Data and Healthcare Data must be used. AI Training Data is collected from different sources such as internet searches, hospital databases, clinical trials, and more. These datasets are used to train machine learning algorithms, allowing them to identify and isolate relevant features from the images. Healthcare Data, on the other hand, is collected from a variety of sources, specifically those related to healthcare. This data can include identifying information about a patient, medications, diagnosis and treatment history, and more. This data is used to inform the interpretation of the potential meaning of any given whole slide image.
For example, AI Training Data can be used to detect and isolate pattern recognition, which is especially useful for medical care where images from different diseases must be compared and analysed. Furthermore, Healthcare Data can be used in a variety of ways including prediction algorithms, trend analysis, and data mining. By combining AI Training Data and Healthcare Data, professionals are able to gain insights on whole slide digital images by identifying and interpreting the relevant features.
In conclusion, when applying AI and analytics to the analysis of whole slide digital images, AI Training Data and Healthcare Data must be used. These strategy enable business professionals to identify and isolate relevant features of the images, which can be used to make more accurate and informed decisions regarding the image in question. In the healthcare field in particular, this data can be invaluable in terms of diagnosis and treatment decisions, as well as predicting future illnesses and diseases. It is clear that leveraging these data sets and AI technology are an invaluable tool for understanding and optimizing whole slide digital images.
Whole slide digital images are invaluable for healthcare professionals in terms of diagnosis and predicting a particular illness or disease. However, due to the fact that the features of the images are generally too small to be seen at a zoomed out scale, this task has been made more difficult over the years. AI and training datasets have made it possible to extract the relevant features of a whole slide image, even at a low resolution, to enable a more comprehensive analysis of these images.
To analyse and make meaningful predictions regarding a particular image, AI Training Data and Healthcare Data must be used. AI Training Data is collected from different sources such as internet searches, hospital databases, clinical trials, and more. These datasets are used to train machine learning algorithms, allowing them to identify and isolate relevant features from the images. Healthcare Data, on the other hand, is collected from a variety of sources, specifically those related to healthcare. This data can include identifying information about a patient, medications, diagnosis and treatment history, and more. This data is used to inform the interpretation of the potential meaning of any given whole slide image.
For example, AI Training Data can be used to detect and isolate pattern recognition, which is especially useful for medical care where images from different diseases must be compared and analysed. Furthermore, Healthcare Data can be used in a variety of ways including prediction algorithms, trend analysis, and data mining. By combining AI Training Data and Healthcare Data, professionals are able to gain insights on whole slide digital images by identifying and interpreting the relevant features.
In conclusion, when applying AI and analytics to the analysis of whole slide digital images, AI Training Data and Healthcare Data must be used. These strategy enable business professionals to identify and isolate relevant features of the images, which can be used to make more accurate and informed decisions regarding the image in question. In the healthcare field in particular, this data can be invaluable in terms of diagnosis and treatment decisions, as well as predicting future illnesses and diseases. It is clear that leveraging these data sets and AI technology are an invaluable tool for understanding and optimizing whole slide digital images.